A colorful Fraunhofer logo on a smartphone is classified as a car (see Figure 1). Our attack demonstrates just how easy it is to manipulate AI-based recognition methods into detecting objects where none exist. This deception could have significant consequences, for example, on the road. These so-called “creation attacks” work with any objects and can be hidden from human view. We are already applying our findings within the Fraunhofer Cluster of Excellence Cognitive Internet Technologies CCIT in the SmartIO project aimed at improving intelligent road intersections.
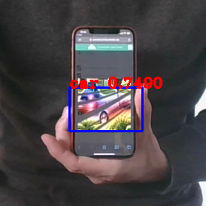
Fig. 1: A colorful Fraunhofer logo on a smartphone is classified as a car.
Attacks from both the inside and the outside
What was the team’s approach? We have been working on so-called “adversarial examples” for several years now. Adversarial examples are preprocessed images or audio files with particular characteristics that confuse AI algorithms into making incorrect classifications. In most cases, the human eye does not recognize these adjustments to the image and audio files — the AI, however, identifies exactly what the attackers want it to detect.
Originally, these attacks were only possible if the input, such as the modified image, was fed directly into the computer. However, these new attacks are even more realistic as they can be carried out remotely, i.e., using images from a camera. Now we have developed a more refined version of this type of attack, known technically as a “physical adversarial attack”. We have drawn on the work of international researchers [1,2,3] to develop an approach that improves the robustness of the attacks.
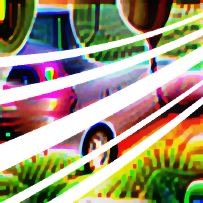
Fig. 2: A so-called ‘Adversarial Example’ leads AI algorithms to wrong classifications.
Algorithm analyzes the neural network
We use a technical algorithm that accurately analyzes the neural network that performs object recognition. Gradient-based methods are used to understand how the input will need to be adjusted in order to fool the neural network. During the optimization process, we created an image that the AI considers to be from the target object’s classification. This results in high detection rates, even if no object is physically present. In order to make the attacks invisible to the human eye, we also considered that manipulations could only occur in a certain area, i.e., in the example shown, only the area around the Fraunhofer logo. It also works in the “real world” as we simulated external changes such as light penetration and color changes when generating the attack pattern. We refreshed the input repeatedly during the generation process: At each step, the attack was tweaked so that it retained its function in different scenarios and under a variety of transformations.
The same procedure works for other target objects: For example, the colorful logo can be transformed into a pedestrian or a street sign instead of a car.
But why is this relevant? Attacks of this type can pose potentially critical safety implications for autonomous vehicles, for example: Attackers can control the driving behavior of another car if they use the image on a smartphone to deceive the object recognition system in the vehicle.
Identifying research requirements and fighting off attacks
We want to use our concrete application example to draw attention to the need for further research into these technologies. The SuKI research project is also investigating other fields of application in addition to those in autonomous vehicles. For example, the project is also focusing on the use of artificial intelligence in speech recognition systems or in the area of access control. More specifically, we are looking at how these kinds of attacks can be blocked by making AI processes resistant to them. The corresponding research results have already been presented at high-level conferences [4-7].
[0] REDMON, Joseph; FARHADI, Ali. Yolov3: An incremental improvement. arXiv preprint arXiv:1804.02767, 2018.
[1] CHEN, Shang-Tse, et al. Shapeshifter: Robust physical adversarial attack on faster r-cnn object detector. In: Joint European Conference on Machine Learning and Knowledge Discovery in Databases. Springer, Cham, 2018. S. 52-68.
[2] ATHALYE, Anish, et al. Synthesizing robust adversarial examples. In: International conference on machine learning. ICML, 2018. S. 284-293.
[3] CARLINI, Nicholas; WAGNER, David. Towards evaluating the robustness of neural networks. In: 2017 ieee symposium on security and privacy (sp). IEEE, 2017. S. 39-57.
[4] SCHULZE, Jan-Philipp; SPERL, Philip; BÖTTINGER, Konstantin. DA3G: Detecting Adversarial Attacks by Analysing Gradients. In: European Symposium on Research in Computer Security. Springer, Cham, 2021. S. 563-583.
[5] SPERL, Philip; BÖTTINGER, Konstantin. Optimizing Information Loss Towards Robust Neural Networks. arXiv preprint arXiv:2008.03072, 2020.
[6] DÖRR, Tom, et al. Towards resistant audio adversarial examples. In: Proceedings of the 1st ACM Workshop on Security and Privacy on Artificial Intelligence. 2020. S. 3-10.
[7] SPERL, Philip, et al. DLA: dense-layer-analysis for adversarial example detection. In: 2020 IEEE European Symposium on Security and Privacy (EuroS&P). IEEE, 2020. S. 198-215a
Additional Information
Authors
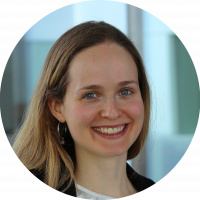
Karla Pizzi
Karla Pizzi has been working as a research fellow at Fraunhofer AISEC since 2018 following her studies in mathematics, political science and computer science.
She is currently working in the Cognitive Security Technologies research department, with a particular focus on adversarial examples to protect artificial intelligence systems from being manipulated.
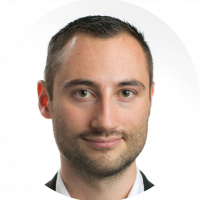
Jan-Philipp Schulze
Jan-Philipp Schulze joined Fraunhofer AISEC as a research fellow in January 2019 after having studied electrical engineering and information technology at ETH Zurich. He is also completing his PhD in computer science at TU Munich. His research work in the Cognitive Security Technologies research department focused on anomaly detection and adversarial machine learning.
Contact: presse@aisec.fraunhofer.de