AI – All that a machine learns is not gold
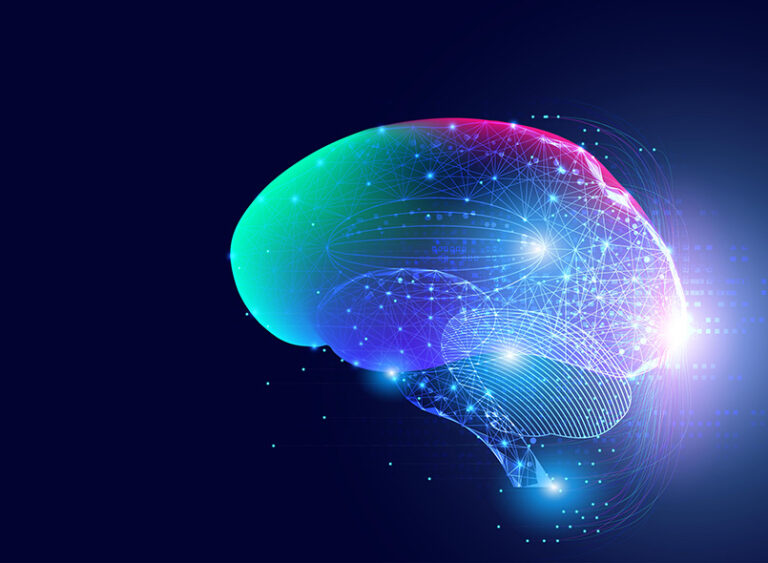
Machine learning is being hailed as the new savior. As the hype around artificial intelligence (AI) increases, trust is being placed in it to solve even the most complex of problems. Results from the lab back up these expectations. Detecting a Covid-19 infection using X-ray images or even speech, autonomous driving, automatic deepfake recognition — all of this is possible using AI under laboratory conditions. Yet when these models are applied in real life, the results are often less than adequate. Why is that? If machine learning is viable in the lab, why is it such a challenge to transfer it to real-life scenarios? And how can we build models that are more robust in the real world? This blog article scrutinizes scientific machine learning models and outlines possible ways of increasing the accuracy of AI in practice.