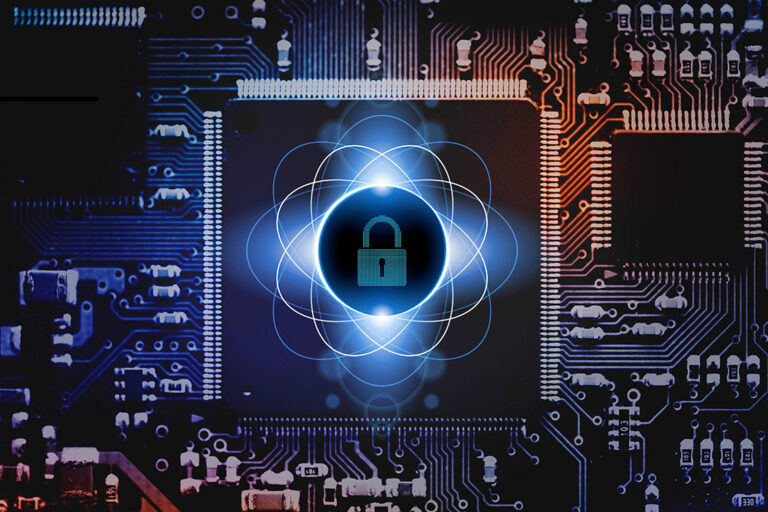
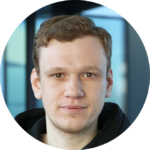
Fortifying Cryptography with Impeccable Circuits: Impeccable Keccak Explained
Cybersecurity threats are evolving, and cryptographic implementations face growing risks from fault injection attacks. Fraunhofer AISEC’s research introduces Impeccable Keccak, a new approach to secure SPHINCS+, a post-quantum cryptography digital signature scheme that has been standardized by NIST in 2024. By leveraging impeccable circuits and ensuring active security, this represents a new approach to fault-resilient cryptography.